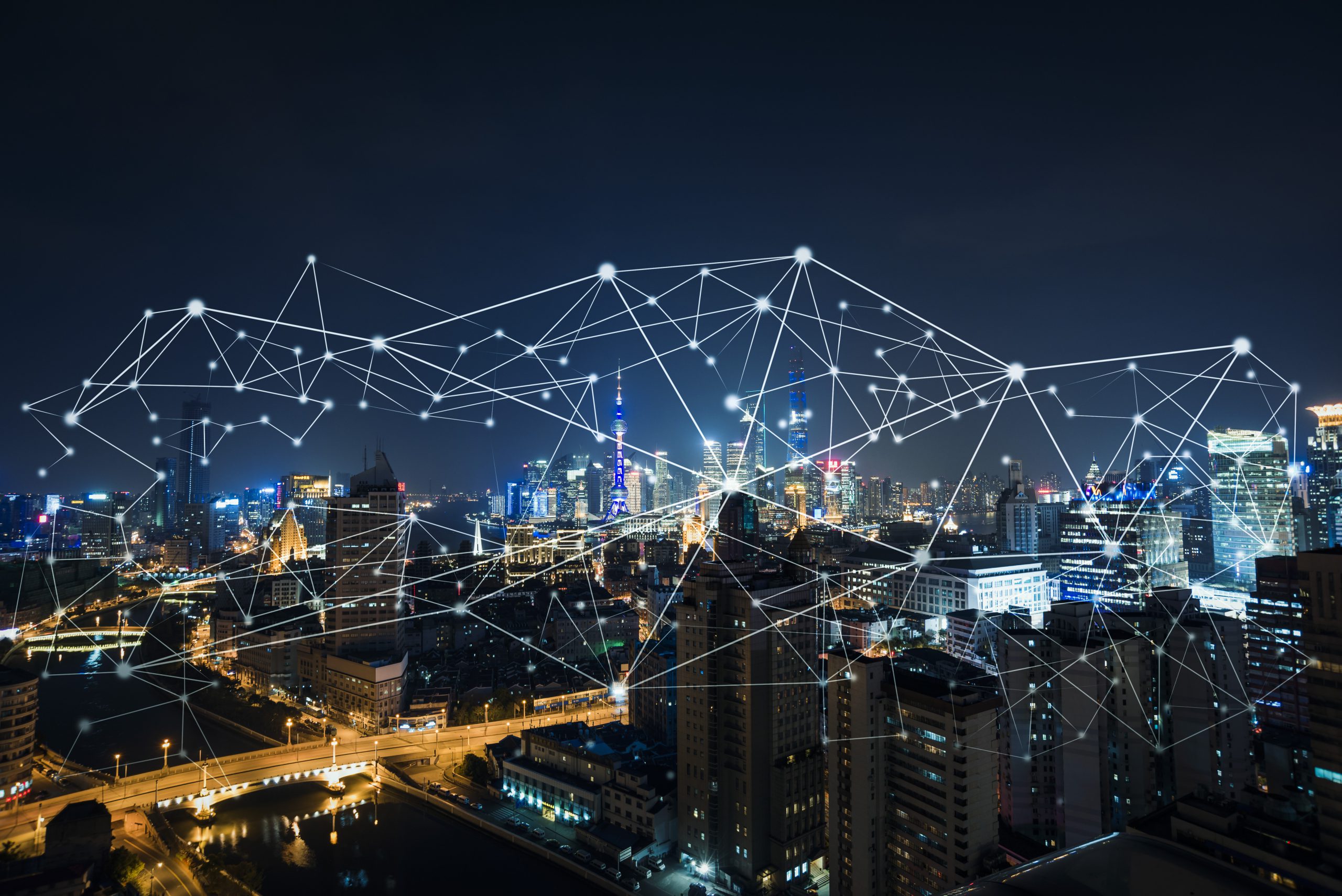
Overview
The cross-cutting theme on Biostatistics and Data Science aims to provide statistical support and develop novel quantitative methods to enable researchers to probe the rich data resources available at the Centre, to analyse multiple complex data sources in a coherent and robust manner, and to reveal the complex interactions and pathways between exposures and outcomes in environment and health studies.
Our research in this area includes the development of statistical methodology and innovative data analytics, anchored in the Bayesian hierarchical modelling paradigm and machine learning, to improve statistical inference. We employ flexible, spatio-temporal semi- and non-parametric models for large complex data from environmental and epidemiological studies and multi-omic high-throughput platforms, and strategies to address the computational challenges of high-dimensional inference and estimation. Such methods are particularly relevant for environment-health assessments and surveillance studies.
Theme Lead
Prof. Marta Blangiardo
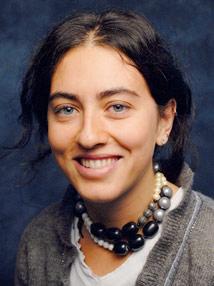
Professor of Biostatistics
Theme Lead: Biostatistics and Data Science
Dept. of Epidemiology and Biostatistics, School of Public Health
View papers for Professor Marta Blangiardo
MRC Centre Themes:
Biostatistics and Data Science – Theme Lead
Cohorts and Data Resources
Molecular Signatures and Disease Pathways
Research areas:
Principal Team
Prof. Marc Chadeau-Hyam
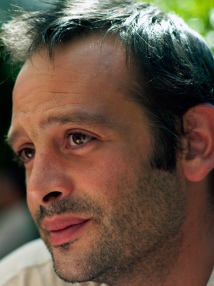
Professor in Computational Epidemiology & Biostatistics
Dept. of Epidemiology and Biostatistics, School of Public Health
View papers for Professor Marc Chadeau-Hyam
Category:
Prof. Tim Ebbels
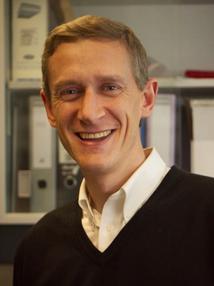
Professor of Biomedical Data Science
Department of Metabolism, Digestion and Reproduction
View papers for Professor Tim Ebbels
MRC Centre Themes:
Biostatistics and Data Science
Molecular Signatures and Disease Pathways
Research areas:
Prof. Klea Katsouyanni
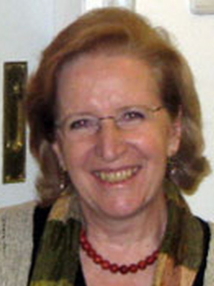
Professor of Public Health
Environmental Research Group, School of Public Health
View papers for Dr Klea Katsouyanni
MRC Centre Themes:
Environmental Exposures
Cohorts and Data Resources
Research areas:
Dr Monica Pirani
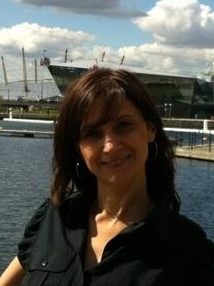
Lecturer in Biostatistics
Dept. of Epidemiology and Biostatistics, School of Public Health
View papers for Dr Monica Pirani
MRC Centre Themes:
Biostatistics and Data Science
Cohorts and Data Resources
Research areas:
Dr Dragana Vuckovic
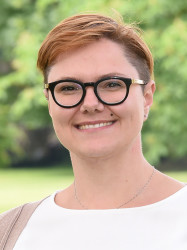
Lecturer in Computational Epidemiology and Biostatistics
Dept. of Epidemiology and Biostatistics, School of Public Health
View papers for Dr Dragana Vuckovic
MRC Centre Themes:
Molecular Signatures and Disease Pathways
Biostatistics and Data Science
Research areas:
Dr Verena Zuber
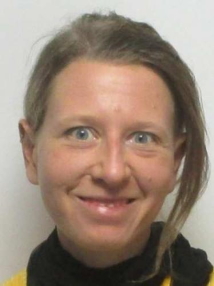
Lecturer in Biostatistics
Dept. of Epidemiology and Biostatistics, School of Public Health
View papers for Dr Verena Zuber
Category:
Associated Team
Dr Daniela Fecht
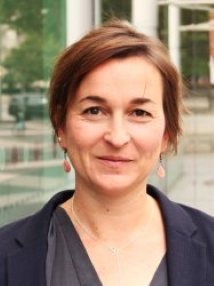
Lecturer in Geospatial Health
Dept. of Epidemiology and Biostatistics, School of Public Health
View papers for Dr Daniela Fecht
MRC Centre Themes:
Healthy Cities, Healthy People
Biostatistics and Data Science
Research areas:
Dr Fred Piel
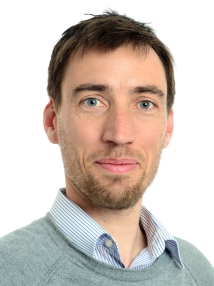
Senior Lecturer in Spatial Epidemiology
Joint Training Programme Director
Dept. of Epidemiology and Biostatistics, School of Public Health
View papers for Dr Fred Piel
MRC Centre Themes:
Biostatistics and Data Science
Cohorts and Data Resources
Research areas:
Key Projects and Papers
• Spatio-temporal methods for 1) understanding the interrelations between climate change, local environmental conditions and arboviral disease dynamics in Brazil (Wellcome Trust grant; PI: Pirani), as well as 2) to quantify the temperature-related respiratory disease burden in England and Wales (MRC Centre funded fellowship).
• Statistical methods to account for spatial uncertainty in small-area data, in particular population census data, and to visualise uncertainty from multiple sources. Collaboration with Emory University (Lance Waller) and Harvard University (Nancy Krieger). (Overall PI: Waller, ICL PI: Piel).
• Comparison of statistical profiling and data analytics for exposome data, including how to include interactions in high dimensional profiling. We devised a multivariate normal approach to analyse exposome data generated using complex study designs with multiple observations per participant and applied it to EXPOsOMICS data. We proposed a series of partial least squares (PLS) models to explore the multivariate effect of exposure mixtures on inflammatory markers.
• Development of the Metabolome Wide Significance Level as an approach to correct for multiple testing in metabolome-wide association studies and a new method for power and sample size calculations for metabolomic studies. We also contributed to the EU-funded PhenoMeNal programme cloud-based infrastructure for computational metabolomics.
• We also have a strong interest in causal inference, in particular how genetic information can be used as an instrumental variable in the Mendelian randomization paradigm to infer causal effects of high-dimensional exposures on outcomes of public health interest.
• Pirani M, Mason AJ, Hansell AL, Richardson S, Blangiardo M. A flexible hierarchical framework for improving inference in area-referenced environmental health studies. doi: 10.1002/bimj.201900241Biometrical Journal. 2020;62:1650-1669
• Itzkowitz N, Gong X, Atilola G, Konstantinoudis G, Adams K, Jephcote C, Gulliver J, Hansell A, Blangiardo Met al., 2023, Aircraft noise and cardiovascular morbidity and mortality near Heathrow Airport: a case-crossover study, Environment International, Vol: 177, Pages: 1-9, ISSN: 0160-4120 doi:10.1016/j.envint.2023.108016
• Zuber, V., Colijn, J. M., Klaver, C. & Burgess, S. Selecting likely causal risk factors from high-throughput experiments using multivariable Mendelian randomization. Nat. Commun. 11, 29 (2020). doi:10.1038/s41467-019-13870-3
• Levin, M. G. et al. Prioritizing the Role of Major Lipoproteins and Subfractions as Risk Factors for Peripheral Artery Disease. Circulation (2021). doi:10.1161/circulationaha.121.053797
• Karimi M, Castagné R, Delpierre C, Albertus G, Berger E, Vineis P, et al. Early-life inequalities and biological ageing: a multisystem Biological Health Score approach in Understanding Society. Journal of Epidemiology and Community Health. 2019:jech-2018-212010 doi:10.1136/jech-2018-212010
• Chadeau-Hyam M, Bodinier B, Vermeulen R, Karimi M, Zuber V, Castagne R, et al. Education, biological ageing, all-cause and cause-specific mortality and morbidity: UK biobank cohort study. EClinicalMedicine. 2020;29-30:100658 doi:10.1016/j.eclinm.2020.100658
• Peluso, Alina, Robert Glen, and Timothy M. D. Ebbels. 2021. 'Multiple-testing correction in metabolome-wide association studies', BMC Bioinformatics, 22: 67. doi: 10.1186/s12859-021-03975-2
• Jendoubi, T., and T. M. D. Ebbels. 2020. 'Integrative analysis of time course metabolic data and biomarker discovery', BMC Bioinformatics, 21: 11. doi:10.1186/s12859-019-3333-0
• Tzoulaki, I., R. Castagne, C. L. Boulange, I. Karaman, E. Chekmeneva, E. Evangelou, T. M. D. Ebbels, M. R. Kaluarachchi, M. Chadeau-Hyam, D. Mosen, A. Dehghan, A. Moayyeri, D. L. S. Ferreira, X. Guo, J. I. Rotter, K. D. Taylor, M. Kavousi, P. S. de Vries, B. Lehne, M. Loh, A. Hofman, J. K. Nicholson, J. Chambers, C. Gieger, E. Holmes, R. Tracy, J. Kooner, P. Greenland, O. H. Franco, D. Herrington, J. C. Lindon, and P. Elliott. 2019. 'Serum metabolic signatures of coronary and carotid atherosclerosis and subsequent cardiovascular disease', European Heart Journal, 40: 2883-96. doi:10.1093/eurheartj/ehz235
• Konstantinoudis G, Padellini T, Bennett J, Davies B, Ezzati M, Blangiardo Met al., 2020, Long-term exposure to air-pollution and COVID-19 mortality in England: a hierarchical spatial analysis, Environment International, ISSN: 0160-4120 doi:10.1016/j.envint.2020.106316
• Davies B, Parkes B, Bennett J, Fecht D, Blangiardo M, Ezzati M, Elliott Pet al., 2021, Community factors and excess mortality in first wave of the COVID-19 pandemic in England, Nature Communications, ISSN: 2041-1723 doi:10.1038/s41467-021-23935-x
• Ponsford, M. J. et al. Cardiometabolic Traits, Sepsis, and Severe COVID-19: A Mendelian Randomization Investigation. Circulation (2020). doi:10.1161/CIRCULATIONAHA.120.050753
• Gill, D. et al. ACE inhibition and cardiometabolic risk factors, lung ACE2 and TMPRSS2 gene expression, and plasma ACE2 levels: a Mendelian randomization study. medRxiv 2020.04.10.20059121 (2020). doi:10.1101/2020.04.10.20059121
• Zuber, V. et al. Leveraging genetic data to elucidate the relationship between Covid-19 and ischemic stroke. medRxiv : the preprint server for health sciences (2021). doi:10.1101/2021.02.25.21252441
• Chadeau-Hyam M, Bodinier B, Elliott J, Whitaker MD, Tzoulaki I, Vermeulen R, et al. Risk factors for positive and negative COVID-19 tests: a cautious and in-depth analysis of UK biobank data. Int J Epidemiol. 2020;49(5):1454-67. doi: 10.1093/ije/dyaa134
• Elliott J, Bodinier B, Whitaker M, Delpierre C, Vermeulen R, Tzoulaki I, et al. COVID-19 mortality in the UK Biobank cohort: revisiting and evaluating risk factors. Eur J Epidemiol. 2021;36(3):299-309. doi:10.1007/s10654-021-00722-y
• Elliott J, Whitaker M, Bodinier B, Riley S, Ward H, Cooke G, et al. Symptom reporting in over 1 million people: community detection of COVID-19. PLOS Medicine. 2021:2021.02.10.21251480. doi:10.1101/2021.02.10.21251480
• Maes M, Pirani M, Booth E, Shen C, Milligan B, Jones K, Toledano M. Benefit of woodland and other natural environments for adolescents' cognition and mental health. Nature Sustainability. 2021 doi:10.1038/s41893-021-00751-1
• Padellini, Tullia, et al. "Time varying association between deprivation, ethnicity and SARS-CoV-2 infections in England: A population-based ecological study." The Lancet Regional Health–Europe 15 (2022) doi:10.1016/j.lanepe.2022.100322
• A Systematic Comparison of Linear Regression-Based Statistical Methods to Assess Exposome-Health Associations. Agier L, Portengen L, Chadeau-Hyam M, Basagaña X, Giorgis-Allemand L, Siroux V, Robinson O, Vlaanderen J, González JR, Nieuwenhuijsen MJ, Vineis P, Vrijheid M, Slama R, Vermeulen R. Environ Health Perspect. 2016 Dec;124(12):1848-1856. doi:10.1289/ehp172
• Blood transcriptional and microRNA responses to short-term exposure to disinfection by-products in a swimming pool. Espín-Pérez A, Font-Ribera L, van Veldhoven K, Krauskopf J, Portengen L, Chadeau-Hyam M, Vermeulen R, Grimalt JO, Villanueva CM, Vineis P, Kogevinas M, Kleinjans JC, de Kok TM. Environ Int. 2018 Jan;110:42-50. doi:10.1016/j.envint.2017.10.003
• Improving Visualization and Interpretation of Metabolome-Wide Association Studies: An Application in a Population-Based Cohort Using Untargeted 1H NMR Metabolic Profiling. Castagné R, Boulangé CL, Karaman I, Campanella G, Santos Ferreira DL, Kaluarachchi MR, Lehne B, Moayyeri A, Lewis MR, Spagou K, Dona AC, Evangelos V, Tracy R, Greenland P, Lindon JC, Herrington D, Ebbels TMD, Elliott P, Tzoulaki I, Chadeau-Hyam M. J Proteome Res. 2017 Oct 6;16(10):3623-3633. doi:10.1021/acs.jproteome.7b00344
• Tan, L. S. L., A. Jasra, M. De Iorio and T. M. D. Ebbels (2017). "Bayesian Inference for Multiple Gaussian Graphical Models with Application to Metabolic Association Networks." Annals of Applied Statistics 11(4): 2222-2251. doi:10.1214/17-AOAS1076
• Power Analysis and Sample Size Determination in Metabolic Phenotyping. Blaise BJ, Correia G, Tin A, Young JH, Vergnaud AC, Lewis M, Pearce JT, Elliott P, Nicholson JK, Holmes E, Ebbels TM. Anal Chem. 2016 May 17;88(10):5179-88. doi:10.1021/acs.analchem.6b00188
• Ebbels TM, Pearce JTM, Sadawi N, Gao J, Glen RC. Chapter 11 - Big Data and Databases for Metabolic Phenotyping. The Handbook of Metabolic Phenotyping. Editor(s): Lindon JC, Nicholson JK, Holmes E. Elsevier, 2019. Pages 329-367.
• Blangiardo M, Cameletti M. Spatial and Spatio-temporal Bayesian Models with R – INLA. Wiley, 2015. doi:10.1002/9781118950203
• R2GUESS: A Graphics Processing Unit-Based R Package for Bayesian Variable Selection Regression of Multivariate Responses. Liquet B, Bottolo L, Campanella G, Richardson S, Chadeau-Hyam M. J Stat Softw. 2016 Jan 29;69(2). doi:10.18637/jss.v069.i02
• A Bayesian mixture modeling approach for public health surveillance. Boulieri A, Bennett JE, Blangiardo M. Biostatistics. 2018 Sep 25. doi: 10.1093/biostatistics/kxy038
• A hierarchical modelling approach to assess multi pollutant effects in time-series studies. Blangiardo M, Pirani M, Kanapka L, Hansell A, Fuller G. PLoS One. 2019 Mar 4;14(3):e0212565. doi:10.1371/journal.pone.0212565
• Bayesian modeling for spatially misaligned health and air pollution data through the INLA-SPDE approach. Cameletti M, Gomez-Rubio V, Blangiardo M. Spatial Statistics 31, 2019 April. doi:10.1016/j.spasta.2019.04.001
• Bayesian spatial modelling for quasi-experimental designs: An interrupted time series study of the opening of Municipal Waste Incinerators in relation to infant mortality and sex ratio. Freni-Sterrantino A, Ghosh RE, Fecht D, Toledano MB, Elliott P, Hansell AL, Blangiardo M. Environ Int. 2019 Jul;128:109-115. doi:10.1016/j.envint.2019.04.009
• Error in air pollution exposure model determinants and bias in health estimates. Vlaanderen J, Portengen L, Chadeau-Hyam M, Szpiro A, Gehring U, Brunekreef B, Hoek G, Vermeulen R. J Expo Sci Environ Epidemiol. 2019 Mar;29(2):258-266. doi:10.1038/s41370-018-0045-x
• Wang, Y., Pirani, M., Hansell, A. L., Richardson, S., & Blangiardo, M. (2019). Using ecological propensity score to adjust for missing confounders in small area studies. Biostatistics, 20(1), 1-16. doi:10.1093/biostatistics/kxx058
• Analysing the health effects of simultaneous exposure to physical and chemical properties of airborne particles. Pirani M, Best N, Blangiardo M, Liverani S, Atkinson RW, Fuller GW. Environ Int. 2015 Jun;79:56-64. doi: 10.1016/j.envint.2015.02.010
• Forlani C., Bhatt S., Cameletti M., Krainski E., Blangiardo M., A Joint Bayesian Space-Time Model to Integrate Spatially Misaligned Air Pollution Data in R-INLA, accepted on Environmetrics, doi:10.1002/env.2644